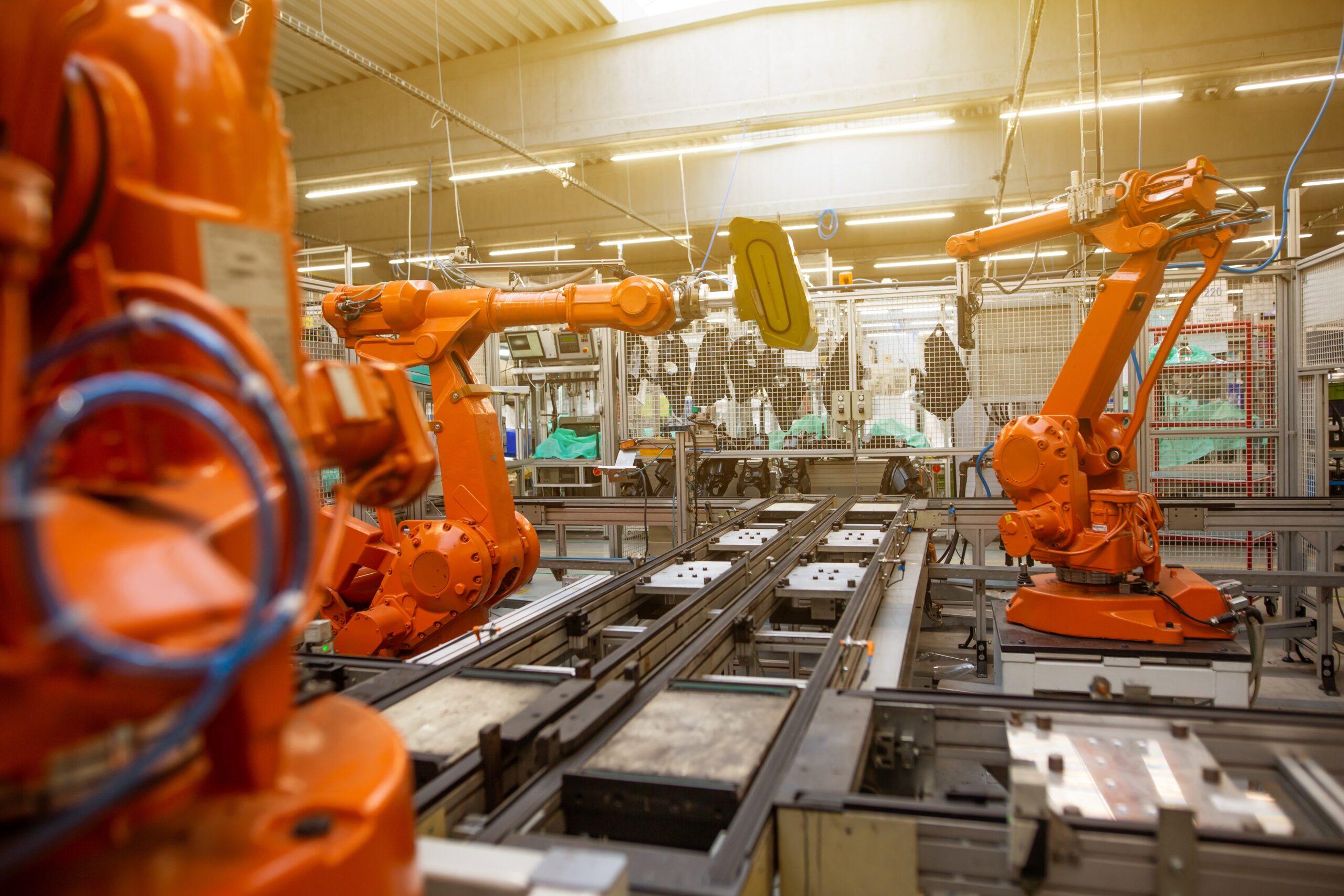
Data Analytics & Business Intelligence in Manufacturing
- Nguyen Huynh
- February 24, 2023
- Blog articles
- 0 Comments
The manufacturing industry has always been a data-rich environment, with vast amounts of data being generated at every stage of the production process. However, until recently, many manufacturers were not taking advantage of this data to drive business decisions. With the advent of data analytics and business intelligence tools, that’s all changing. Manufacturers are now able to leverage data to optimize their operations, improve quality control, and reduce costs.
The amount of data generated by the manufacturing sector can vary depending on the industry and the specific operations of a company. However, in general, the manufacturing sector is known to generate a large volume of data, particularly with the increasing use of sensors, automation, and digital technologies in manufacturing processes. Some examples of data that are typically generated in the manufacturing industry include:
- Production data: This includes information on the quantity, quality, and type of products being produced, as well as information on the production process itself, such as the time taken for each step in the production process.
- Equipment and machine data: This includes data on the performance and maintenance of equipment and machines used in the production process. This can include information on machine uptime, utilization, and downtime, as well as data on equipment failures and maintenance history.
- Quality control data: This includes data on the quality of the products being produced, such as information on defects, scrap rates, and rework rates.
- Inventory data: This includes information on the quantity and location of raw materials, work-in-progress inventory, and finished goods inventory.
- Supply chain data: This includes data on suppliers, transportation, and logistics, such as lead times, order quantities, and delivery performance.
- Energy and environmental data: This includes data on energy consumption, carbon emissions, and other environmental impact factors.
As this data is generated from various sources such as sensors, machines, production lines, supply chain management systems, customer feedback, and other third-party sources, it is usually stored in different formats and locations, including databases, spreadsheets, data warehouses, and data lakes. As a result, many organizations are implementing advanced data management systems to store and manage their data effectively.
These advanced data management systems are designed to handle large amounts of data from multiple sources and make it easy to analyze and extract insights. These systems can include technologies such as data warehouses, data lakes, and other big data platforms.
Data warehouses are designed to collect and store structured data from various sources, such as transactional systems or other databases, and transform it into a format that is optimized for reporting and analysis. These systems are typically managed by IT teams and are used to provide high-performance access to historical data for business intelligence and other analytics applications.
Data lakes, on the other hand, are designed to store large amounts of raw, unstructured, or semi-structured data from a variety of sources, such as IoT sensors or social media feeds. Data lakes are often used to enable advanced analytics and machine learning, which require large amounts of data to train models and make predictions. These systems are often managed by data science or analytics teams and may require specialized skills to extract insights from the data.
Nowadays, advanced data management systems are critical for enabling data analytics and business intelligence in the manufacturing sector. There are several trends in this field that should be on every manufacturer’s radar – if they are to remain competitive. Here are a few:
- Predictive maintenance: Many manufacturers are using machine learning and predictive analytics to anticipate equipment failures before they occur. By analyzing data from sensors and other sources, they can identify patterns that indicate impending problems and take action to prevent downtime.
- Supply chain optimization: Manufacturers are also using data analytics to optimize their supply chains, reducing costs and improving efficiency. By analyzing data on suppliers, inventory, and production processes, they can identify bottlenecks and inefficiencies and make adjustments to improve performance.
- Quality control: Data analytics can also be used to improve product quality and reduce defects. By analyzing data from sensors, cameras, and other sources, manufacturers can identify defects and quality issues and take corrective action.
- Real-time monitoring: Real-time monitoring is becoming increasingly important in the manufacturing sector, allowing manufacturers to monitor production processes and respond quickly to issues. By using sensors and other devices to collect data in real-time, manufacturers can identify issues as they arise and take corrective action before they become major problems.
- Artificial intelligence and machine learning: Finally, many manufacturers are exploring the use of artificial intelligence and machine learning to improve their operations. These technologies can be used to automate processes, improve decision-making, and optimize performance, leading to greater efficiency and productivity.
One example of the use of data analytics in the manufacturing industry comes from a large steel manufacturer in Germany, ThyssenKrupp Steel, which has implemented a predictive maintenance program using machine learning and IoT sensors to detect and address potential issues in their machinery before they result in costly downtime. By analyzing real-time data on machine performance, ThyssenKrupp Steel is able to optimize maintenance schedules and reduce overall maintenance costs.
Or take BMW, the global automotive manufacturer, which has implemented a real-time quality control system using data analytics and machine learning to improve the quality of their production. The system monitors production data, including machine settings, sensor readings, and employee input, to identify patterns and predict potential quality issues. By catching potential problems early, BMW is able to minimize scrap and rework costs, while improving overall quality.
In a third example, General Electric (GE) uses data analytics to optimize the performance of its gas turbines. By collecting and analyzing data on factors such as temperature, pressure, and fuel consumption, GE can identify opportunities to improve the efficiency of its turbines, reducing fuel consumption and emissions, and ultimately improving the bottom line.
These case studies demonstrate the diverse ways in which data analytics and business intelligence can be applied in the manufacturing industry, from predictive maintenance to energy management and performance optimization. By harnessing the power of data, manufacturers can gain valuable insights into their operations, improve efficiency, and reduce costs.
Leave A Comment
You must be logged in to post a comment.